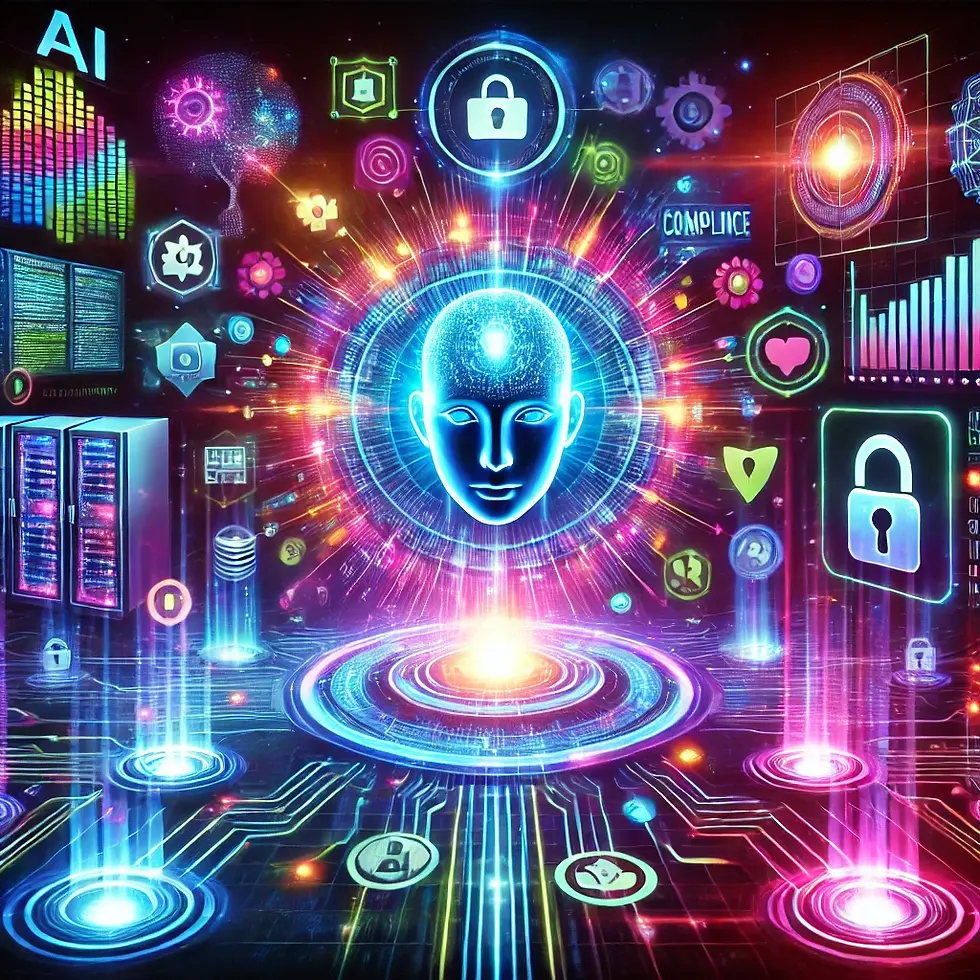
U.S.-based organizations deploying artificial intelligence (AI) systems in the European Union (EU) must now navigate the complexities of the forthcoming EU AI Act, a landmark regulation that came into force only a few months ago, on August 1, 2024.
The Act, which applies extraterritorially, to any company whose AI systems impact EU citizens, introduces strict compliance obligations based on the risk level of AI technologies.
High-risk systems, such as those used in healthcare, recruitment, and biometric identification, are subject to the most stringent governance standards, including transparency, risk management, and data governance. In contrast, low-risk applications face fewer requirements, but companies across the board will need to prepare for a new regulatory landscape.
In this context, robust information governance (IG) practices are essential for ensuring compliance with the EU AI Act. Particularly important are metadata tagging, redundant, obsolete, and trivial (ROT) data removal, and retention-schedule-based records management are key IG strategies that can help organizations manage AI-related data responsibly and meet the Act’s stringent requirements.
These practices not only promote compliance but also improve AI system performance and mitigate legal risks. Real-world case studies demonstrate how these IG strategies can be applied to align with the provisions of the EU AI Act.
The following case studies provide some perspective on this alignment.
AI applications such as robotic process automation are often used in industrial settings for predictive maintenance, which the EU AI Act classifies as minimal-risk AI. While these systems face fewer regulatory burdens than higher-risk activities such as profiling, companies subject to the EU AI Act can still benefit from employing metadata tagging to track and manage the data used in these AI models. For example, by tagging data related to equipment performance and maintenance schedules, organizations can enhance the traceability and reliability of their AI systems. This process helps ensure that any AI-driven decisions made regarding equipment maintenance are based on accurate and up-to-date data, thus minimizing operational risks. Moreover, should the organization ever be required to demonstrate the safety and efficacy of its AI system to regulators or auditors (or in the context of its ESG-reporting obligations), the metadata provides a clear audit trail.
At the other end of the risk spectrum, high-risk AI applications used in healthcare—such as AI-based medical software—are subject to far more stringent requirements under the EU AI Act. These systems must undergo rigorous compliance assessments and maintain transparent documentation of data usage. In this context, metadata tagging becomes an indispensable tool for documenting the source, accuracy, and relevance of the data used to train the AI models. In healthcare settings, for example, this could mean tagging patient data with information about the condition it relates to, the date it was collected, and the outcome of medical interventions. Developing an accurate system for metadata tagging of medical AI systems helps to ensure that these systems can operate on high-quality, relevant data, helping to maintain compliance with the EU AI Act’s requirements for accuracy, robustness, and human oversight.
Another closely related tool is the use of information governance-based strategies to remove redundant, obsolete, and trivial (ROT) data. AI systems in healthcare often rely on vast datasets, including patient records and diagnostic information. Without proper data management, these datasets can become cluttered with irrelevant or outdated information, leading to inaccurate AI-driven outcomes or even outright data hallucinations, and increasing the risk of non-compliance. By implementing and developing ROT removal technologies, healthcare organizations can ensure that their AI systems are trained and operated using only the most relevant and reliable data. This not only improves the performance of the AI system but also ensures that it complies with the EU AI Act’s requirements for data quality and governance.
Another area where ROT-data removal plays a significant role is in the use of AI systems for employment and recruitment—which the EU AI Act also classifies as high-risk. Employers that develop and deploy these AI systems must ensure that they comply with the Act’s requirements for transparency, human oversight, and accuracy, particularly in terms of how they process and analyze personal data related to job candidates. For example, AI-driven recruitment platforms that analyze resumes and filter candidates based on qualifications or previous experience must ensure that the data used is accurate and relevant to the hiring decisions being made. By eliminating redundant or outdated candidate data, organizations can reduce the risk of AI bias or errors and ensure that their recruitment systems comply with the Act’s governance standards.
Retention-schedule-based records management is another IG strategy that plays a vital role in complying with the EU AI Act. One important use case is that of biometric identification systems, which the ACT also categorizes as high-risk. Biometric identification systems rely on sensitive personal data, such as facial recognition or fingerprint data, to function. The EU AI Act stringently regulates how this data is handled, including retention and deletion protocols. Aligning records management practices with a defensible retention schedule helps organizations ensure that biometric data is only retained for as long as necessary and is deleted once it is no longer required. This reduces the risk of retaining sensitive data beyond its legally required period, helping organizations avoid potential privacy penalties.
As the AI landscape continues to evolve, as regulatory scrutiny intensifies, and as organizations continue to rely on and deploy ever-increasing stores of data, the structured use of information governance best practices becomes ever more critical to compliance with laws like the EU AI Act. These tools not only mitigate legal risks but also enhance the overall quality, performance, and trustworthiness of their AI systems and demonstrate to both stakeholders and regulators that you are taking the truth of your AI models seriously.
Comments