Transforming Unstructured Data for AI Success with Information Governance
- Max Rapaport
- Jan 2
- 4 min read
Updated: Jan 5
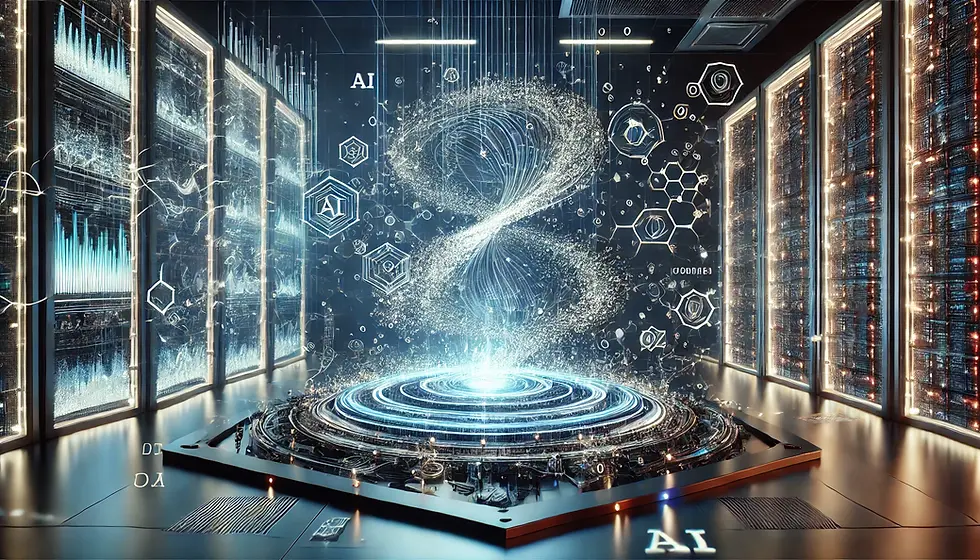
The era of artificial intelligence (AI) promises transformative opportunities, but with those opportunities comes a challenge: managing the vast oceans of unstructured data enterprises generate every day. As highlighted in the recent "Market Momentum Index: AI and Unstructured Data Management" report by AIIM, M-Files, and Deep Analysis, organizations are grappling with the dual pressures of leveraging AI while navigating the complexities of unstructured data management.
This report which surveyed contributions from over 500 U.S.-based enterprises across key industries—including Financial Services, Manufacturing, and Utilities—highlights the current state of AI adoption, the readiness of unstructured data for AI-driven initiatives, and emphasizes the tools and strategies utilized to overcome challenges in security, compliance, and data accuracy.
Unstructured data—the videos, emails, images, and files that don’t fit neatly into a database—is both a treasure trove of insights and a liability. Without a robust information governance (IG) framework, this data can become redundant, obsolete, and trivial (ROT), undermining the reliability of AI models like large language models (LLMs). This post discusses how smart IG practices can turn the tide, transforming ROT into a solid foundation for AI reliability.
The Cost of ROT in an AI-Driven World
Unstructured data now represents 67% of enterprise cloud storage, yet only 4% of organizations report a decrease in the cost of managing it. This disconnect is driven by several challenges:
Volume and Variety: Data exists in myriad formats, from text to audio and video, making discovery and classification difficult.
Data Quality Issues: ROT data accumulates over time, muddying AI initiatives and hampering performance.
Compliance Risks: Sensitive information hidden in unstructured data poses legal and regulatory risks.
Access Governance Challenges: Fragmented access controls increase the risk of breaches and inefficiencies.
Finding solutions to these challenges isn't just a technical issue! It is existential for organizations relying on AI to deliver accurate, reliable results.
Why IG Matters: The AI-IG Connection
The connection between IG and AI is not just complementary—it’s essential. AI, particularly LLM-based models, rely on high-quality, structured, and relevant data to function effectively. Without strong IG practices, unstructured data quickly becomes a liability rather than an asset. Here’s how IG drives AI success:
1. Reducing ROT to Improve AI Accuracy
Unstructured data often includes ROT content that can mislead AI models or skew their outputs. For example, outdated documents or duplicated data can introduce inconsistencies, resulting in AI "hallucinations" or incorrect predictions. IG frameworks focus on identifying and defensibly deleting ROT data, ensuring that only accurate and current datasets are used for training and analysis.
2. Enhancing Data Quality for Reliable AI Outputs
AI thrives on precision, but unstructured data is often plagued by incomplete, irrelevant, or low-quality content. Information governance strategies prioritize data curation, ensuring datasets meet critical quality benchmarks, such as freshness, accuracy, and relevancy. By systematically validating and improving data, organizations can avoid costly errors and improve the reliability of AI outcomes.
3. Ensuring Transparency Through Data Lineage
A lack of transparency in data origins—known as data lineage—can erode trust in AI models. Without understanding where data comes from, how it’s been transformed, or who has accessed it, verifying AI outputs becomes nearly impossible. IG practices like lineage tracking, or the process of documenting and visualizing the origins, transformations, and destinations of data throughout its lifecycle, help organizations to create a clear map of data’s journey, promoting compliance, accountability, and confidence in AI decisions.
4. Building Ethical and Compliant AI Models
AI systems are increasingly subject to regulations like the EU’s AI Act, GDPR, and CCPA, which require clear governance over how data is collected, stored, and used. IG practices embed compliance controls at every stage of data management, from discovery and classification to access and retention. These measures prevent sensitive data exposure, mitigate legal risks, and ensure AI models operate within ethical and legal boundaries.
5. Safeguarding Data Security and Privacy
Security breaches and privacy violations are significant concerns for organizations using unstructured data in AI pipelines. IG frameworks emphasize robust access controls, encryption, and data anonymization, protecting sensitive information throughout its lifecycle. For AI models, this means safer training environments and minimized risks of exposing personal or confidential data.
6. Streamlining AI-Ready Data Management
One of the biggest barriers to effective AI adoption is siloed and inaccessible data. IG creates unified data governance processes that make unstructured data discoverable, cataloged, and accessible across departments. This eliminates inefficiencies and ensures that AI systems have a seamless pipeline of high-quality data.
7. Strengthening AI Trust with Stakeholders
Stakeholder trust is paramount for AI adoption, especially in sectors like financial services and healthcare. A strong IG foundation demonstrates that data is managed responsibly, ethically, and transparently. This trust is critical not only for internal buy-in but also for regulatory bodies, customers, and partners.
8. Aligning AI with Business Objectives
Finally, IG ensures that AI initiatives are aligned with broader organizational goals. By leveraging IG to prioritize relevant and impactful data, businesses can ensure that their AI investments drive measurable outcomes, whether in customer insights, operational efficiencies, or competitive advantages.
By addressing these critical areas, information governance doesn’t just complement AI—it enables it. The integration of IG practices ensures that unstructured data is transformed from a chaotic liability into a strategic asset, laying the foundation for AI systems that are accurate, ethical, and trustworthy. With this synergy, organizations can unlock the true potential of AI while navigating an increasingly complex data landscape.
Comments